Theory and Practice of Historical Data Versioning
The Case of the Venice and Lausanne Census and Cadastral Datasets
large dataset, epistemological challenges, census transcription
Introduction
Among digital approaches to historical data, we can identify various types of scholarship and methodologies practiced in creating transcription corpora of documents that are valuable for research, specifically quantitative analyses. Demographic, cadastral, and geographic sources are particularly notable for their transcripts, which are instrumental in generating datasets that can be used or reused across different disciplinary contexts.
From a disciplinary perspective, it is essential to observe certain trends that have fundamentally transformed the methodology of making historical data accessible and studying them. These trends extend the usability of historical data beyond the individual scholar’s interpretation to a broader community. The creation of datasets that can then serve again to different communities of study is increasingly practiced by scholars; the data, before their interpretations, acquire an autonomous value, an important authorial legitimacy. Just as is already currently practiced in the natural sciences, the provision of datasets becomes crucial to history as well. Computational approaches, however, follow trends common to other fields, and the increasing use of artificial intelligence to extract, refine, realign, and understand historical data compels the integration of clear and well-described protocols to inform the datasets that are created.
Lausanne and Venice Census Datasets
The first example are the IIIF (International Image Interoperability Framework) protocols. These protocols allow the community to employ computational approaches to use these sources as a foundation for refining techniques to extract the embedded information. Open sources are made possible by heritage institutions that recognize the added value of studying their collections with computational approaches, thus transforming them into indispensable objects of research for interdisciplinary and ever-expanding community. Another significant trend is the creation of datasets through the extraction of information contained in sources by diverse communities from various disciplinary fields. These communities might be interested in the computational methodologies, extraction techniques, or the historical content of the extracted data. The field of Digital Humanities, addressing the latter aspect, has effectively highlighted that each element extracted from historical documents should, whenever possible, be directly referenced to the document from which it was taken. This can be achieved through IIIF-compatible datasets. Moreover, the methods for extracting, processing, and normalizing the data must always be thoroughly explained and are subject to scholarly critique and evaluation. Emphasizing the importance of maintaining the link between extracted data and original documents ensures transparency and accuracy, thus enhancing the credibility and usability for digital historical research.
When publishing a historical dataset, adhering to a methodology that consistently maintains the link to the original source is crucial. Wherever possible, the source document itself should be made accessible. Additionally, the methods used to extract historical information must be clearly stated. This transparency allows for subsequent corrections, refinements, and improvements to the dataset by other researchers, even a posteriori (Maryl 2023). This means that datasets can be corrected and refined, particularly when normalizing entity names such as places or people. In this context, we are moving beyond the traditional reliance on the author and their citation. Every piece of information valuable for historical reconstruction should be validated by tracing its publication genesis: from the original source, through its interpretation, to its final dataset form (Traub 2020).
Specifying the version of the data released and the one being worked on for improvements is essential. When these datasets are studied with quantitative analysis approaches, any profound transformation of the dataset—such as an improved version through OCR correction—can lead to a re-evaluation of all existing interpretations (Bürgermeister 2020). This ensures that the resulting historical narratives can be re-examined and updated accordingly.
Many projects that handle historical data, such as datasets containing information about people or places, follow established pipelines. These pipelines typically involve an initial transcription phase, which can be manual, semiautomatic, or automatic (using machine learning or artificial intelligence), followed by OCR (Optical Character Recognition) verifications and, finally, entity alignment with existing dictionaries or the creation of specific ontologies for the extracted entities.
The new potentials afforded by the latest computational techniques —whether based on manual annotation, automatic machine learning, or OCR extraction through AI— position us on the brink of what we may soon call the data deluge of information drawn from historical sources (Kaplan and Lenardo 2017). Pioneering approaches to harnessing this deluge are already proliferating (Gibbs and Owens 2013). This vast quantity of mined data makes manual exploration impractical, necessitating an approach where computational methods facilitate asking questions, analyzing answers, and reformulating inquiries.
These new methods will partially reshape historical approaches, requiring engagement with extensive datasets of varied origins. The validation of data extraction will become a critical task within both human and computational pipelines. This raises the question of the accuracy of information in the extracted datasets, making the extraction and correction process itself crucial.
Several key elements emerge in this context. First, there is a distinction between creating datasets accurate enough to locate results—such as names or places—in specialized search engines and creating datasets for precise theoretical and historical analysis. While these elements are related, they operate on different temporal scales.
Processing and extracting millions of transcript segments is a computationally intensive task. In the case of the “Names of Lausanne” project (EPFL-CROSS project n. 20212), part of the larger Lausanne Time Machine initiative, dealing with census sources containing millions of text segments, after an initial phase of manual annotation training, it is essential to rely on computational approaches. Although the results do not always perfectly reflect the original text and may contain spelling errors, they are often sufficient for an elastic search or distance-approximative navigation.
RESTful search and analytics engine capable of addressing a growing number of use cases is particularly well suited for managing and querying large datasets (Gormley and Tong 2015). It enables full-text search, structured search, and analysis, providing a robust framework for handling large amounts of historical data (Neudecker and Antonacopoulos 2016). The flexibility of elastic search means that it can handle complex queries and provide fast search results, even in datasets that contain inconsistencies or errors. This capability is essential to enable initial navigation and interaction with the huge volumes of data generated by historical sources, facilitating further detailed analysis, and possibly enabling collaborative approaches to correction.
This means that millions of units of information can be made “searchable,” even if they do not correspond to a fully curated dataset. Through further approaches –such as contributory appeals for correction or progressive improvements in transcription techniques– these datasets can eventually produce “structured” and verified datasets, as understood in the historical sciences. Consequently, it will become increasingly essential to trace the computational origin of extracted items. This involves documenting the methods by which the data were processed and specifying the version of the dataset to which they correspond, recognizing that these versions are subject to continuous improvement.
By applying these principles to historical datasets, we can ensure that the data extracted is not only accessible and traceable, but also continuously refined and validated through collaborative efforts and methodological advances.
In “Names of Lausanne”, Petitpierre et al. (2023) automatically extracted 72 census records of Lausanne. The complete dataset covers a century of historical demography in Lausanne (1805-1898), corresponding to 18,831 pages, and nearly 6 million cells (Figure 1).
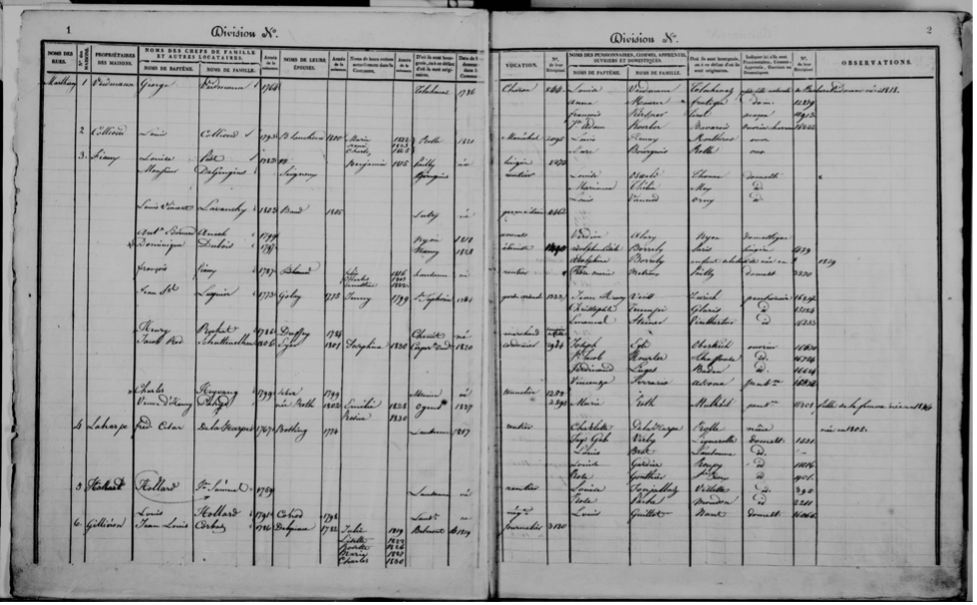
The structure and informational wealth of censuses have also provided an opportunity to develop automatic methods for processing structured documents. The processing of censuses includes several steps, from identifying text segments to restructuring information as digital tabular data through Handwritten Text Recognition and the automatic segmentation of the layout using neural networks (Petitpierre, Kramer, and Rappo 2023). The data are structured in rows and columns, where each row corresponds to a distinct household.
The historical information is particularly rich, including the composition of the household, children, place names, servants, housemates, as well as the occupations, municipalities of origin, and dates of birth of all persons mentioned. The documents present considerable reading difficulties, and the liability of the first version of the dataset extracted, combining Handwritten Text Recognition (HTR) with pre-processing and post-correction strategies based on supervised language models, achieved a character error rate of 3.44%. These improvements result in high-quality data suitable for research in demographic and social history. The data are already searchable, and can later be queried using string distance heuristics, or statistical methodologies to deal with noise and uncertainty (Figure 2). The goal is to make these transcribed datasets, produced using automatic approaches, available in a collaborative annotation tool that will allow a progressively larger number of mentions to be corrected manually, and use the corrections to progressively reprocess and improve the corpus.
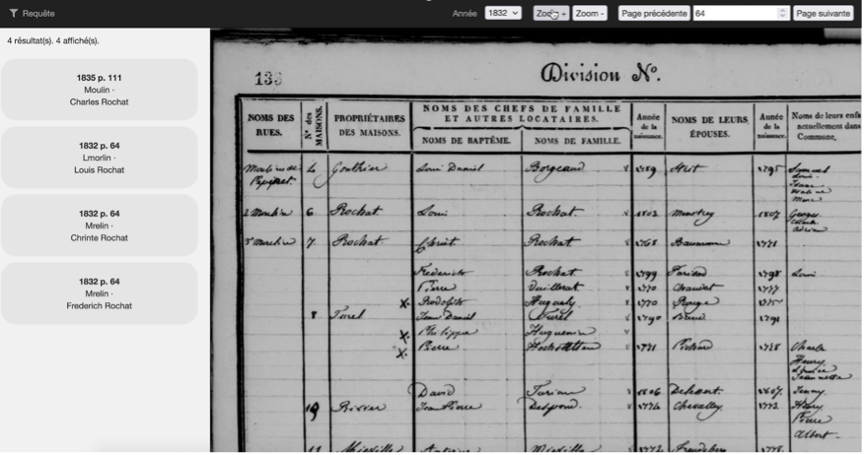
In the case of “Parcels of Venice” (FNS project - 185060), land records from the Napoleonic land register (1808) were analyzed (Figure 3). They contain a parcel number with some associated attributes, such as owner, function, and area, which are linked to plans on which the geometries of the parcels are drawn, and can be reassembled on a map of Venice (Lenardo et al. 2021).
Some 23,428 lines of documents were manually extracted and then analyzed, which included mentions of owners, functions, and house numbers, as well as other correspondences. In this case, the mentions will also be entered into a text search engine that can filter and combine different fields, but there was a need to standardize some items, for example, family surnames and functions, in order to proceed with quantitative historical analyses. The proprietary text entries in the dataset are too noisy for reliable analysis, due to the lack of standard delimiters, missing surnames, frequent use of the term “above” (“as above”), and other inconsistencies. Additional information, such as family relationships or origin details, complicates entries. No general algorithm can accurately correct and standardize these entries; therefore, a manual standardization approach was used. This involved manually editing all 23,428 entries, aided by a customizable Jupyter Notebook tool, to apply specific corrections across the dataset (Musso 2023). The goal was different; it was not only to make the data searchable but also to make it analyzable, particularly regarding ownership. The classification of owners into three types —City of Venice, Institution, and Person— was carried out in a three-step process, resolving 23,395 entries. The unresolved entries did not match any of the predefined categories.
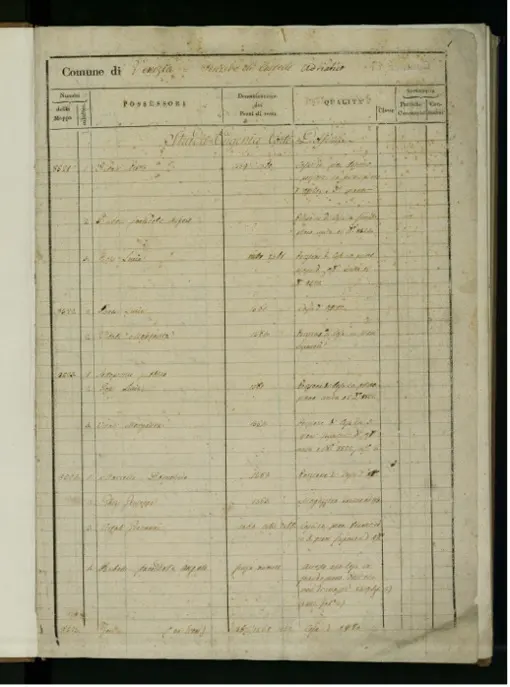
For named entity recognition, a “people” dataset was created, linking individuals with their parcels based on disambiguated person mentions from the Sommarioni records. A merging protocol was employed to consolidate entries with the same name and additional matching attributes into unique person objects, resulting in a comprehensive and standardized dataset ready for detailed analysis. The dataset navigation interface thus indicates not only the authorship of the manual transcription, where present, but also whether the displayed data correspond to a semi- or fully automatic extraction or entrusted even partially to the language models (Figure 4). It will also be possible, via GitHub, to trace the extraction production Jupyter notebook 1. This will allow the user, whether the general public or scholars to clarify the genesis of the data represented.
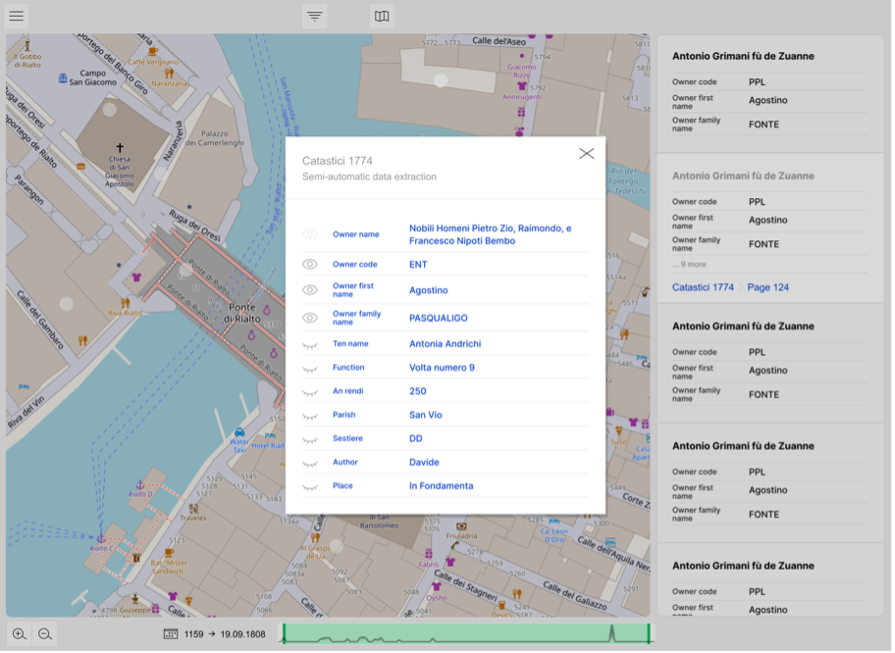
An initial version of this dataset will be published and will already allow some key aspects of land tenure to be studied, however, not all fields of information have been standardized, which is why we can build on this version later to improve machine reading and produce new corrections.
These projects underscore the transformative impact of OCR and HTR technologies, coupled with language models, on the extraction and correction processes of historical documents. The challenge lies in consistently documenting the computational process origins within datasets, ensuring users can evaluate the reliability of the data. As the quality of data extraction and transcription improves, new historical narratives may emerge, emphasizing the critical need to track data versions and correct older datasets to prevent potential inaccuracies.
References
Footnotes
Reuse
Citation
@misc{di_lenardo2024,
author = {di Lenardo, Isabella and Petitpierre, Rémi and Rappo, Lucas
and Guhennec, Paul and Musso, Carlo and Mermoud-Ghraichy, Nicolas},
editor = {Baudry, Jérôme and Burkart, Lucas and Joyeux-Prunel,
Béatrice and Kurmann, Eliane and Mähr, Moritz and Natale, Enrico and
Sibille, Christiane and Twente, Moritz},
title = {Theory and {Practice} of {Historical} {Data} {Versioning}},
date = {2024-09-13},
url = {https://digihistch24.github.io/submissions/456/},
doi = {10.5281/zenodo.13907752},
langid = {en},
abstract = {This article discusses the evolution of digital approaches
to historical data, particularly in the creation and use of
transcripted corpora extracted from demographic, cadastral, and
geographic sources. These datasets are crucial for quantitative
analyses across various disciplines. The emergence of computational
techniques, including manual annotation, machine learning, and OCR,
has led to a significant influx of historical data, challenging
traditional methods of analysis. This paper emphasizes the
importance of maintaining data versioning and transparency, enabling
corrections and refinements over time. It highlights projects like
“Names of Lausanne” and “Parcels of Venice,” which use advanced
technologies to create searchable and analyzable datasets from
historical censuses and cadastral records. These projects illustrate
the critical role of versioning and proper data management in
preserving the integrity and usability of historical data, allowing
for continuous improvements and new historical insights.}
}